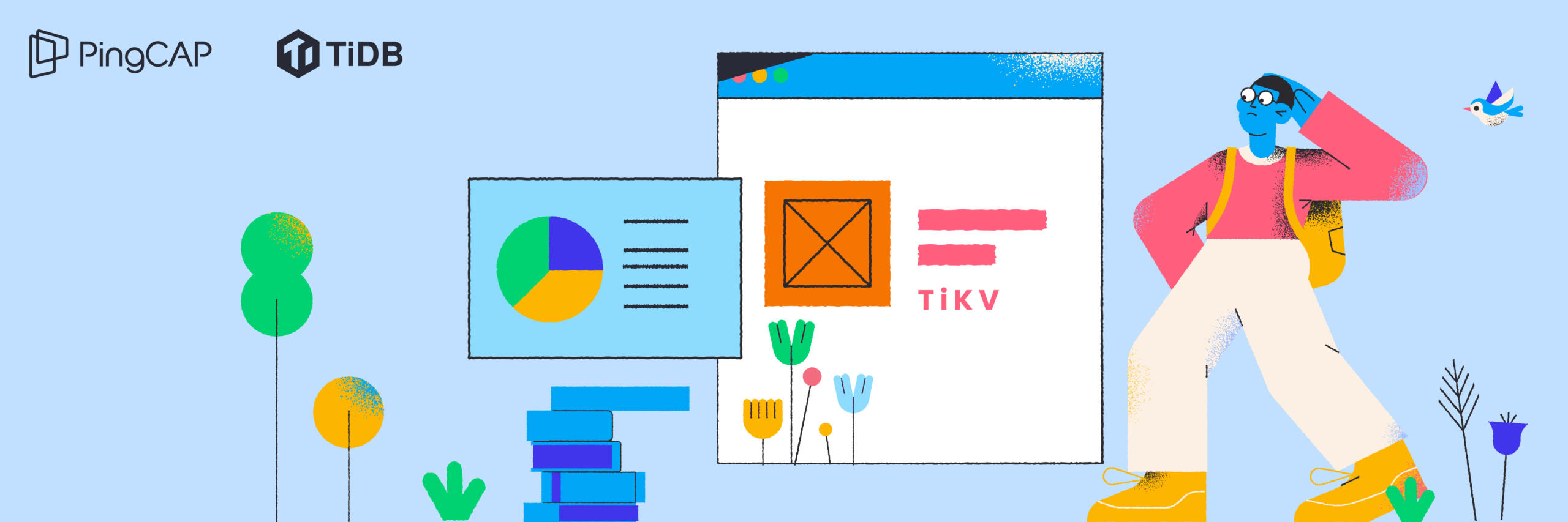
TiDB is an open-source, distributed, NewSQL database that supports Hybrid Transactional and Analytical Processing (HTAP) workloads. It is MySQL compatible and features horizontal scalability, strong consistency, and high availability.
TiDB includes TiKV, a row-oriented, key-value storage engine. TiKV plays a key role in high availability (HA). Data from TiDB is split into several Regions, the smallest data unit for replication and load balancing. Then, each Region is replicated three times and distributed among different TiKV nodes. The replicas for one Region form a Raft group.
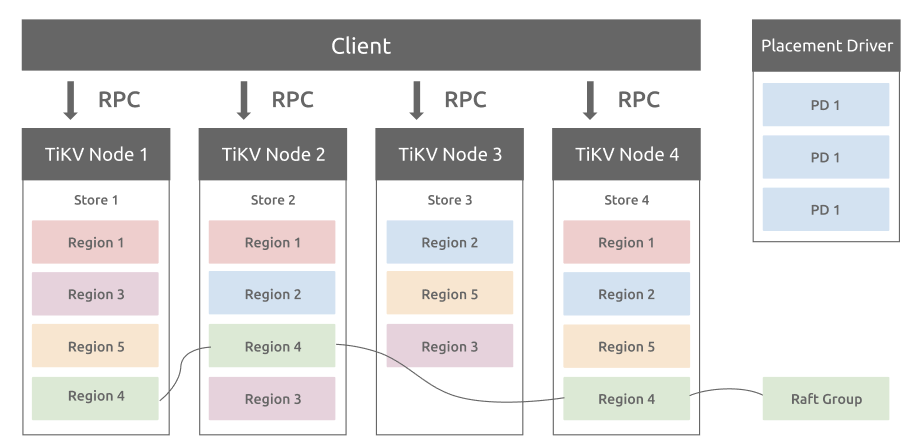
TiKV architecture
There are two parts of data that need to be stored: the data itself and the Raft logs. Two RocksDB instances are used to store the data in each TiKV node. In earlier versions of TiDB, RocksDB stores the Raft logs, where they are converted into key-value pairs. However, using RocksDB to store Raft logs caused write amplification (WA), which created huge amounts of I/O. TiDB introduced Raft Engine—a log-structured embedded storage engine for multi-Raft logs in TiKV.
In this article, we will dive deep into the Raft Engine—why we need it, how we design and implement it, and how it benefits performance.
Pain points of using RocksDB to store Raft logs
The biggest pain point of using RocksDB to store Raft logs is the large amount of I/O that’s generated. In RocksDB, this I/O comes from two places.
First, in RocksDB, key-value pairs are first inserted into a Write Ahead Log (WAL), and then the data are written to the RocksDB MemTable. When the data in MemTable reaches a certain size, RocksDB flushes the content into a Sorted String Table (SST) file in the disk. This means that the same information is written to the disks twice.
RocksDB also has write amplification (WA). WA is the ratio between the amount of data written to storage devices and the amount of data written by users. Key-value (KV) stores which are based on log-structured merge trees (LSM-trees) have long been criticized for their high WA due to frequent compactions. As the dataset increases, so does the depth of the LSM-tree and the overall WA. Increased WA consumes more storage bandwidth, competes with flush operations, and eventually slows application throughput.
The huge amount of RocksDB I/O becomes an obvious issue when deploying TiDB in the cloud environment or using TiDB Cloud. The reasons are two-fold. First, cloud vendors usually charge each I/O. In addition the amount of RocksDB I/O fluctuates a lot, which may impact the quality of service (QoS).
Raft Engine design
Inspired by BitCask, we designed and implemented Raft Engine, a persistent embedded storage engine with a log-structured design. It is built for TiKV to store Multi-Raft logs. The figure below shows a simplified Raft Engine architecture.
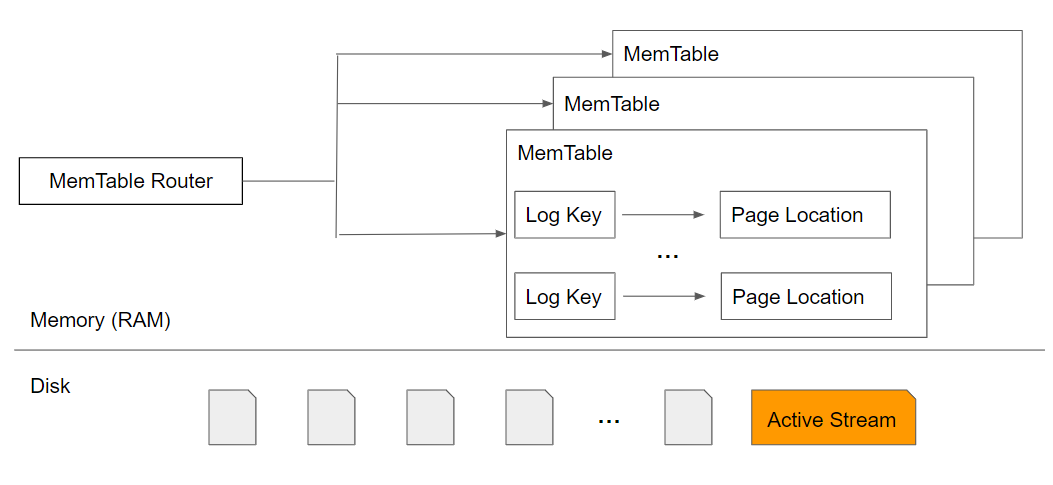
The simplified Raft Engine architecture
On the disk, write requests, both the key and the actual data, are sequentially written to the active append-only log file. When a configurable threshold is reached, a new file is created.
MemTable is an in-memory hash table. It contains log keys and the page location (offset) of that key. The MemTable is an index for the storage on disk. The MemTable is split into several parts. When a read or write request comes in, the MemTable Router directs the request to the corresponding MemTable.
Read a log file
When reading a log file, the reader first visits the MemTable Router to locate the corresponding MemTable. The reader gets the page location of the log from the MemTable based on the log key. Once that information is available, the engine performs one disk read from the file on the disk.
Insert a log file
To insert a new record, the engine:
- Appends the entry and key value to the active data file (stream)
- Creates an entry in the MemTable
These two operations are performed atomically. Inserting a new record only involves one disk write and no disk seek.
Raft Engine also supports lz4 compression. You have the option of compressing the data before it’s written to the disk. This compression can help reduce the write I/O. The records in the MemTable are not compressed.
Update a log file
Updating a log file is similar to inserting a new KV pair. The difference is that, instead of creating a new entry in MemTable, the existing record is updated with the new page location. The old value in the disk is now dangling and will be removed during garbage collection in the purge phase.
Delete a log file
To delete a log file, the engine performs two atomic operations. It:
- Appends a new entry in the active data stream with the value equalling a tombstone
- Deletes the MemTable entry
Just like when the log file was updated, the old record is now dangling and will be removed during garbage collection in the purge phase.
Raft Engine recovery
When Raft Engine is restarted, the engine reads the Log Key on the disk and builds the MemTable based on it. This is different from BitCask. In BitCask, there are hint files stored on the disk, which hold the necessary data for recovery. We deprecated the hint file in Raft Engine, because it causes extra write I/O in the background. Based on our internal benchmarked testing, Raft Engine still recovers very fast. When the data stored on Raft Engine is less than 10 GB, It usually takes less than 2 seconds to recover.
Purge
The purge phase permanently deletes old entries on the disks. Purge is similar to the compaction in the RocksDB. However, the purge in Raft Engine is much more efficient.
During the compaction phase in RocksDB, all data files must be read into memory, combined, and then flushed to the disk. Because Raft Engine has an index for all records in MemTable, it only needs to read the up-to-date data, combine them into a file, and then flush them to the disk. Obsolete pages are then abandoned.
The user determines when data is purged The Raft Engine consolidates and removes its log files only when the user calls the purge_expired_files() routine
. By default, TiKV calls the purge function every 10 seconds.
The Raft Engine also sends useful feedback to the user. Each time the purge_expired_files()routine
is called, Raft Engine returns a list of Raft Groups that hold particularly old log entries. Those log entries block garbage collection and should be compacted by the user.
Design considerations
Why does the Raft Engine meet our needs better than the RocksDB?
As discussed earlier, in our scenario RocksDB generates huge amounts of I/O. The values in RocksDB are sorted by their keys. This provides very good performance when conducting range queries; however, in our case we don’t need range queries. Sorting the values based on keys inevitably leads to huge write amplification.
Raft Engine does not have this feature, so it generates less I/O.
Why are there multiple MemTables?
When data is read from or written to a MemTable, the table must be locked. Subsequent requests must wait in the queue until the current operation finishes. When multiple MemTables are used, if two requests access different MemTables, they do not block each other.
Performance evaluation
Now, let’s compare the performance of RockDB and the Raft Engine using the TPC-C benchmark. We set up a TiDB cluster as follows:
- The TiDB and Placement Driver (PD) servers are on Amazon Web Services (AWS) c5.4xlarge instances.
- The TiKV servers are on r5.4xlarge instances with 1 TB gp3 EBS volumes.
- For TPC-C benchmarking, we use 5,000 warehouses.
- We run the experiments with 50 and 800 threads.
Each experiment runs for two hours.
50 threads
TpmC | Client latency (ms) | CPU (%) | I/O (MB/s) | IOPS | |
RocksDB | 28,954.7 | 50th percentile: 44.090th: 54.5 95th: 60.899th: 79.7 99.9th: 151.0 | 420 | Write: 98.0Read: 24.0 | Write: 1,850 Read: 400 |
Raft Engine | 30,146.4 | 50th: 41.990th: 52.4 95th: 58.799th: 79.7 99.9th: 142.6 | 430 | Write: 75.0Read: 25.0 | Write: 1,850Read: 450 |
Performance comparison between RocksDB and Raft Engine with 50 threads
800 threads
TpmC | Client latency (ms) | CPU (%) | I/O (MB/s) | IOPS | |
RocksDB | 54,846.9 | 50th percentile: 402.790th: 570.495th: 604.099th: 805.399.9th: 1,073.7 | 850 | Write: 209.0 Read 54.0 | Write: 1,750Read: 1,200 |
Raft Engine | 56,020.5 | 50th: 402.790th: 570.495th: 604.099th: 671.199.9th: 838.9 | 750 | Write: 129.0Read: 49.0 | Write: 1,700 Read: 1,200 |
Performance comparison between RocksDB and Raft Engine with 800 threads
Based on the data above, when we compare Raft Engine to TiKV with RocksDB, Raft Engine:
- Improves QPS by up to 4%
- Reduces tail latency by 20%
- Reduces write I/O is reduced by 25% ~ 40%
- Reduces CPU usage by 12% under heavy workloads
Want to learn more?
TiDB Cloud is a fully managed Database-as-a-Service (DBaaS) that brings you everything great about TiDB on the cloud. Ready to give TiDB Cloud a try? TiDB Cloud Developer Tier is now available! You can run a TiDB cluster for free for one year on Amazon Web Services.
Try TiDB Cloud Free Now!
Get the power of a cloud-native, distributed SQL database built for real-time
analytics in a fully managed service.
To stay updated on TiDB Cloud news, make sure to follow us on Twitter. You can also check our website for more information about our products and services or request a demo.
Keep reading:
- Improve Performance and Data Availability with Elastic Block Store
- How We Reduced Multi-region Read Latency and Network Traffic by 50%
- TiDB Operator Source Code Reading (V): Backup and Restore
(Edited by Fendy Feng, Tom Dewan)
Experience modern data infrastructure firsthand.
TiDB Cloud Dedicated
A fully-managed cloud DBaaS for predictable workloads
TiDB Cloud Serverless
A fully-managed cloud DBaaS for auto-scaling workloads