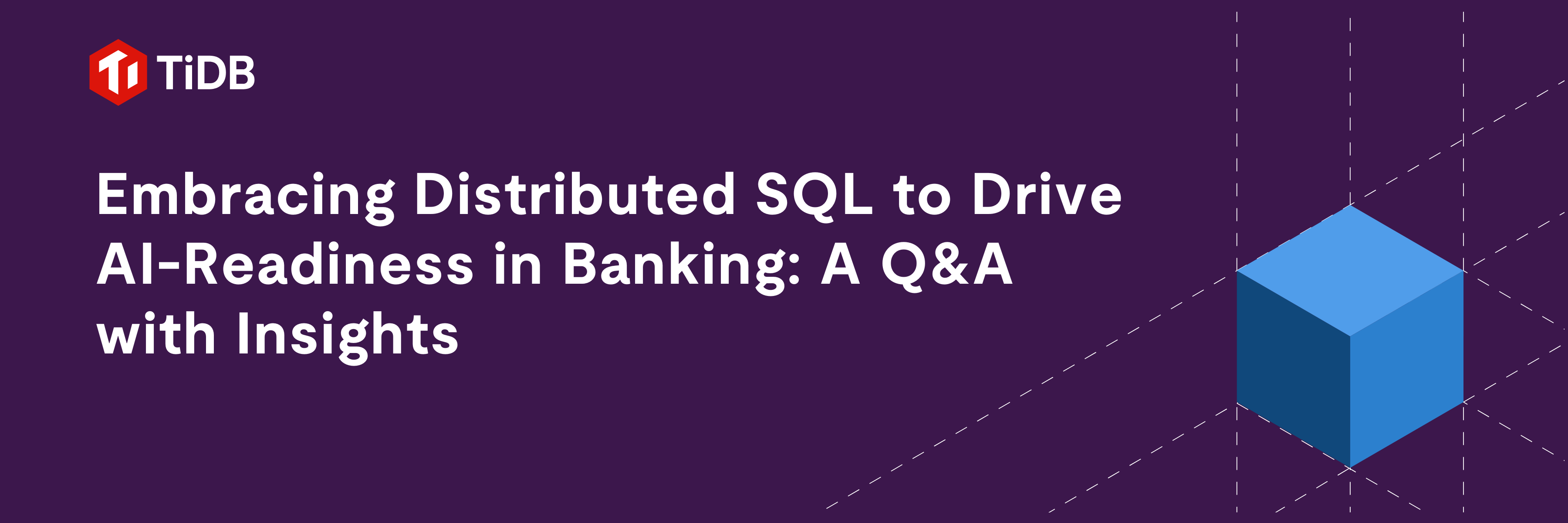
Financial services are rapidly transforming to adopt AI, digitize operations, and enhance customer experiences, yet many banks struggle with outdated legacy data systems that limit AI adoption.
At the Silverlake Axis Customer Connect Conference (SACCC), held from October 17 to 20, 2024, in Da Nang, Vietnam, industry leaders focused on modernizing banking infrastructure. C-suite executives, board members, and experts gathered to explore financial innovation, with sessions spotlighting the role of databases in achieving AI readiness. Experts from PingCAP and Silverlake Axis discussed how distributed SQL, particularly TiDB, can overcome legacy limitations, empower seamless scaling, and deliver real-time insights.
During the session, the Silverlake Axis Chairman surprised the panel with a challenging question on how banks can address disparate requirements when modernizing their data architecture, adding depth to the conversation on banking’s AI-driven future.
In this Q&A blog, we capture the key insights from Terry Purcell, Chief Optimizer Architect at TiDB, who shared his expertise on how distributed SQL can unlock AI’s potential in banking. Dr.Ashish Kakar, IDC Financial Insights Asia/Pacific Research Director, moderated the discussion.
In this blog, we’ve condensed the information into questions asked by Dr.Ashish and Terry Purcell’s answers in first person.
Why do legacy data systems pose a challenge for AI adoption in banking?
I believe legacy data systems pose a significant challenge to AI adoption in banking. I draw this perspective from an extensive background in database engineering. I spent 18 years working on mainframe Db2 development at IBM, where I led the query optimizer team. Over those years, I saw the strengths of these legacy systems but also their limitations. Systems like Db2 and Oracle are built on codebases that are around 45 years old. This age makes them inherently slow to innovate. They weren’t designed to handle today’s AI-driven environments, with their scale, speed, and integration demands.
One major obstacle to AI adoption in banking is fragmentation within these legacy architectures. Many banks operate hundreds of disparate applications. This creates a highly disjointed data landscape. The result is data silos, where information is spread across multiple platforms. Each platform requires separate management and maintenance. This setup adds significant complexity and operational inefficiency. It becomes nearly impossible for banks to consolidate data effectively for real-time insights.
My experience with Db2 has made me keenly aware of the need for a simpler, more adaptable data architecture. Over the past 12 years, distributed SQL has emerged as a critical innovation. It was developed from the ground up to address modern requirements. Unlike legacy databases, distributed SQL offers massive scalability and flexibility. It also simplifies the data landscape. With distributed SQL, banks can reduce the number of disparate systems. This move helps them shift toward a more streamlined, future-proof architecture.
I believe that shifting to distributed SQL offers a path forward. This transition allows banks to overcome the limitations of their legacy systems. By adopting a distributed SQL architecture, financial institutions can consolidate their data infrastructure. They can reduce complexity and pave the way for real-time data processing. This shift ultimately enables AI-driven insights.
How can distributed SQL help banks overcome these challenges?
Distributed SQL offers a solution to the limitations of legacy systems, especially in banking, where stability and regulatory compliance are crucial. Banks face more stringent requirements than other industries. Maintaining high availability and consistent data access is essential for them. Distributed SQL helps manage these challenges more effectively.
I’ve seen firsthand how this approach works. A large U.S.-based social media company struggled with data fragmentation across disparate systems. Their developers had to manually piece together data from various sources, including OLAP systems, big data environments, AI models, and OLTP databases. The costs of this fragmentation were significant. They needed a unified solution to present a consistent data view to developers.
Distributed SQL solved this issue by enabling horizontal scalability. It integrates different data workloads—both transactional and analytical—within one platform. This approach simplifies the data landscape, allowing banks to consolidate their infrastructure. Instead of relying on multiple systems, they can use one unified database architecture. For developers, this makes working with data more accessible and speeds up innovation.
Interestingly, the demand for large-scale data integration and real-time processing often originates from non-banking sectors. Innovations driven by these industries are advancing distributed SQL. I’m confident these advancements will benefit banks, helping them future-proof their systems. Distributed SQL addresses financial institutions’ needs by simplifying architecture, consolidating databases, and providing the scalability required for AI applications. This allows banks to streamline operations, gain more value from their data, and stay ahead of industry demands.
Are there real-world examples where distributed SQL has been successfully implemented in banking?
There are several significant use cases where distributed SQL, specifically TiDB, has proven valuable across the banking sector, particularly in Asia:
- China Construction Bank (CCB): In China, CCB has fully embraced distributed SQL by running its core banking applications on TiDB. This includes more than 120 applications, leading to substantial performance improvements. Other Chinese banks, like WeBank and Bank of Hangzhou, have also migrated from MySQL and Oracle to TiDB, enhancing their core banking, mobile, and batch-processing operations. Chinese banks are seeing a 50% performance improvement in mobile banking performance.
- Indonesian Bank: Recently, a major Indonesian bank migrated its mobile banking application from MariaDB to TiDB, achieving a 50% boost in mobile banking performance and a remarkable 10x improvement in backup and recovery speeds. This upgrade enabled the bank to meet regulatory compliance requirements that were previously unattainable.
- North America: While banks in North America have been slower in adopting distributed SQL, there has been progress. For instance, a Linklarge credit card merchant recently completed a proof of concept (POC) with TiDB, resulting in a 10x increase in batch processing performance after moving off MySQL. Additionally, fintech companies like Plaid are more willing to innovate, finding distributed SQL solutions beneficial for scaling and improving data consistency.
The Asia-Pacific region is leading the way in distributed SQL adoption, while the U.S. is gradually catching up.
How do distributed SQL solutions compare scalability, AI-readiness, and resilience to NoSQL databases?
Distributed SQL outperforms NoSQL databases in several key areas. Distributed SQL is designed for resilience, with robust architecture options like multi-AZ (availability zone) and multi-cloud backup and recovery scenarios. This flexibility ensures high availability and data protection, crucial for critical banking applications where downtime and data loss are simply unacceptable.
When it comes to scalability, distributed SQL has reached a maturity level that surpasses NoSQL databases. While NoSQL solutions can work for niche use cases or non-critical applications, they often struggle to handle high-volume transactional workloads alongside complex analytics. In contrast, distributed SQL supports Hybrid Transactional and Analytical Processing (HTAP), allowing banks to manage real-time transactions and analytics within the same system.
Given the resilience of legacy systems, can distributed SQL truly replace them for core banking applications?
I’ve long recognized that the resilience of legacy systems like Db2 and Oracle has been one of their key strengths, and understandably, this makes banks hesitant to replace them. These systems have matured over decades and have features that ensure stability, data integrity, and reliability—essential for core banking functions. However, I believe distributed SQL has now reached a level of maturity where it can match, and in some cases even exceed, the resilience of these traditional databases.
For banks looking to adopt distributed SQL, I suggest starting with non-core or “fringe” applications. This could include mobile banking, AI-based analytics, or customer-facing applications. Adopting distributed SQL for these functions allows banks to gain hands-on experience with the technology and build confidence in its capabilities without immediately overhauling its core systems. As distributed SQL proves itself in these less critical areas, banks can gradually expand its use to their core functions.
Silverlake Chairman Question: Given the diverse requirements and the shift towards unified data and content, how can databases meet the evolving expectations for AI in banking?
I acknowledge the complexity of the challenge here—disparate requirements often lead to fragmented database architectures. This fragmentation makes it challenging to innovate, especially within monolithic systems that are not built for the modern demands of AI and data integration.
To me, simplicity is crucial. Distributed SQL can help by reducing the number of separate databases and minimizing data copies, making it far easier to deliver a seamless application experience. Innovation is challenging when you’re dealing with so many disjointed systems. The more unified the architecture, the more we can focus on delivering real value rather than just managing complexity.
I also believe that while databases are critical to this transformation, they shouldn’t be the focal point. Instead, they should enable seamless integration and support AI-driven insights without becoming a bottleneck. I’m optimistic about the ongoing innovations in distributed SQL. This technology is already showing promise in meeting these evolving expectations, simplifying data architectures, and enhancing the overall user experience.
Distributed Databases: The Future of Banking
The Fireside Chat at the Silverlake Axis Customer Connect Conference offered an insightful look into the future of banking data architecture. Thanks to Dr. Ashish Kakar for expertly moderating the discussion with Terry Purcell on overcoming legacy system limitations and leveraging distributed SQL.
As the discussion highlighted, banks must adapt to modern demands with a data architecture that is resilient, scalable, and ready for AI integration. Distributed SQL, particularly TiDB, offers a path forward by addressing these challenges with cutting-edge solutions that can future-proof the financial sector.
To explore the topics discussed above, download our IDC InfoBrief, which offers insights on transforming banking data architecture with distributed SQL and real-world case studies.
Experience modern data infrastructure firsthand.
TiDB Cloud Dedicated
A fully-managed cloud DBaaS for predictable workloads
TiDB Cloud Serverless
A fully-managed cloud DBaaS for auto-scaling workloads