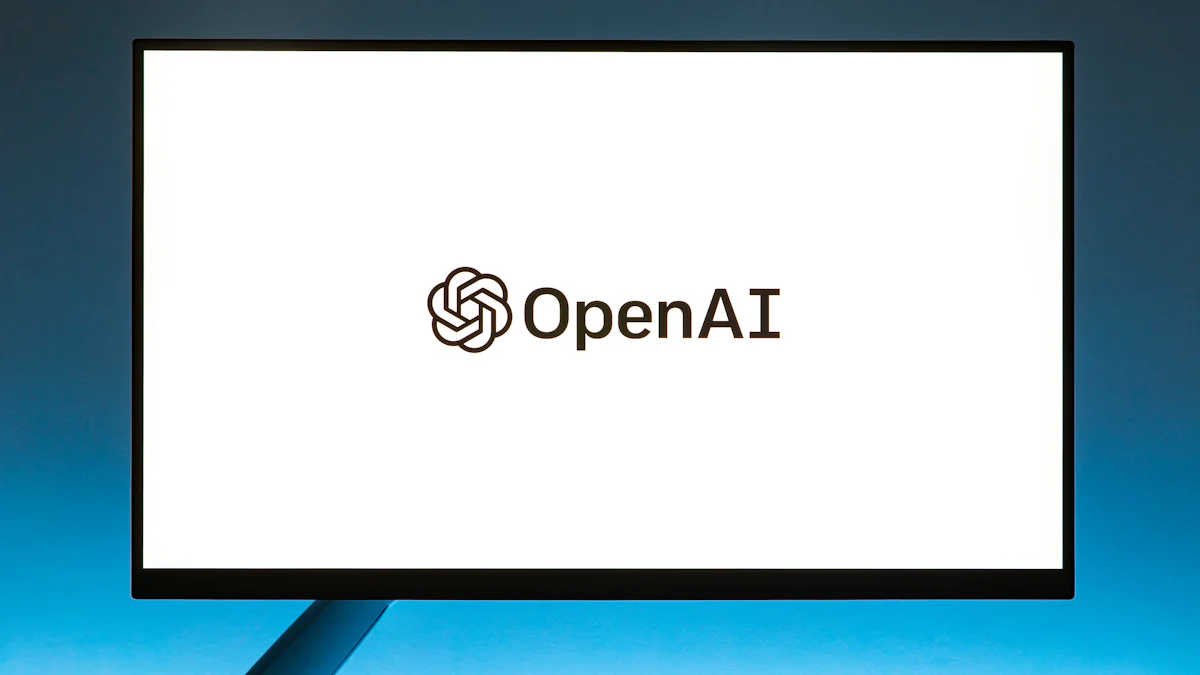
OpenAI, founded in 2015, has been at the forefront of AI research, aiming to develop technologies that benefit humanity. One of its groundbreaking contributions is the OpenAI CLIP model, which bridges the gap between computer vision and natural language processing. By associating images with textual descriptions, CLIP has revolutionized how we understand and interact with visual data. This blog aims to provide a comprehensive understanding of the OpenAI CLIP model, highlighting its significance and applications in the AI landscape.
Understanding the Basics of OpenAI CLIP
What is OpenAI CLIP?
Definition and Overview
OpenAI CLIP (Contrastive Language-Image Pre-training) is a groundbreaking model developed by OpenAI that bridges the gap between computer vision and natural language processing. By leveraging a vast dataset of 400 million image-text pairs, CLIP learns to associate images with their textual descriptions. This enables it to perform tasks like zero-shot classification, where it can label unseen images without requiring additional training on new datasets. The model’s ability to understand and interpret visual data through natural language makes it a versatile tool in the AI landscape.
Key Features and Innovations
OpenAI CLIP stands out due to several key features and innovations:
-
Zero-Shot Learning: CLIP can classify images into categories it has never seen before, using only natural language descriptions.
-
Multimodal Capabilities: It integrates both visual and textual data, allowing for more nuanced understanding and interaction.
-
Efficient Training: CLIP’s training approach reduces the need for extensive labeled datasets, making it quicker and more cost-effective to fine-tune for specific tasks.
-
Versatile Applications: From image retrieval and content moderation to enabling multi-modal machine learning techniques, CLIP’s applications are vast and varied.
The Development of CLIP
Background and Motivation
The development of OpenAI CLIP was driven by the need to create a model that could understand and interpret the rich connections between visual and textual data. Traditional models often required large, labeled datasets and were limited to specific tasks. OpenAI aimed to overcome these limitations by developing a model that could generalize across various domains and perform well even with minimal training on new datasets.
Research and Development Process
The research and development process for OpenAI CLIP involved training the model on a massive dataset of 400 million image-text pairs. This self-supervised learning approach allowed the model to learn from the natural co-occurrence of images and text on the internet. The team employed contrastive learning techniques to align the representations of images and their corresponding textual descriptions in a shared embedding space. This innovative approach enabled CLIP to achieve impressive performance on a wide range of tasks without the need for task-specific training.
How CLIP Works
Contrastive Learning
At the core of OpenAI CLIP is contrastive learning, a technique that trains the model to differentiate between matching and non-matching image-text pairs. By maximizing the similarity between correct pairs and minimizing it for incorrect ones, CLIP learns to create robust embeddings that capture the semantic relationships between images and text. This method allows the model to generalize well to new, unseen data, making it highly effective for zero-shot learning tasks.
Language-Image Pre-training
OpenAI CLIP employs a unique pre-training strategy that involves jointly training a text encoder and an image encoder. The text encoder processes textual descriptions, while the image encoder processes visual data. Both encoders map their respective inputs into a shared embedding space, where semantically related images and text are closely aligned. This pre-training approach enables CLIP to understand and generate meaningful associations between visual and textual information.
Model Architecture
The architecture of OpenAI CLIP consists of two main components:
-
Text Encoder: Typically based on transformer models like GPT, the text encoder converts textual descriptions into high-dimensional vectors.
-
Image Encoder: Using convolutional neural networks (CNNs) or vision transformers, the image encoder transforms visual data into corresponding vectors.
These vectors are then aligned in a shared embedding space, enabling the model to perform tasks that require understanding the relationship between images and text.
Applications of CLIP
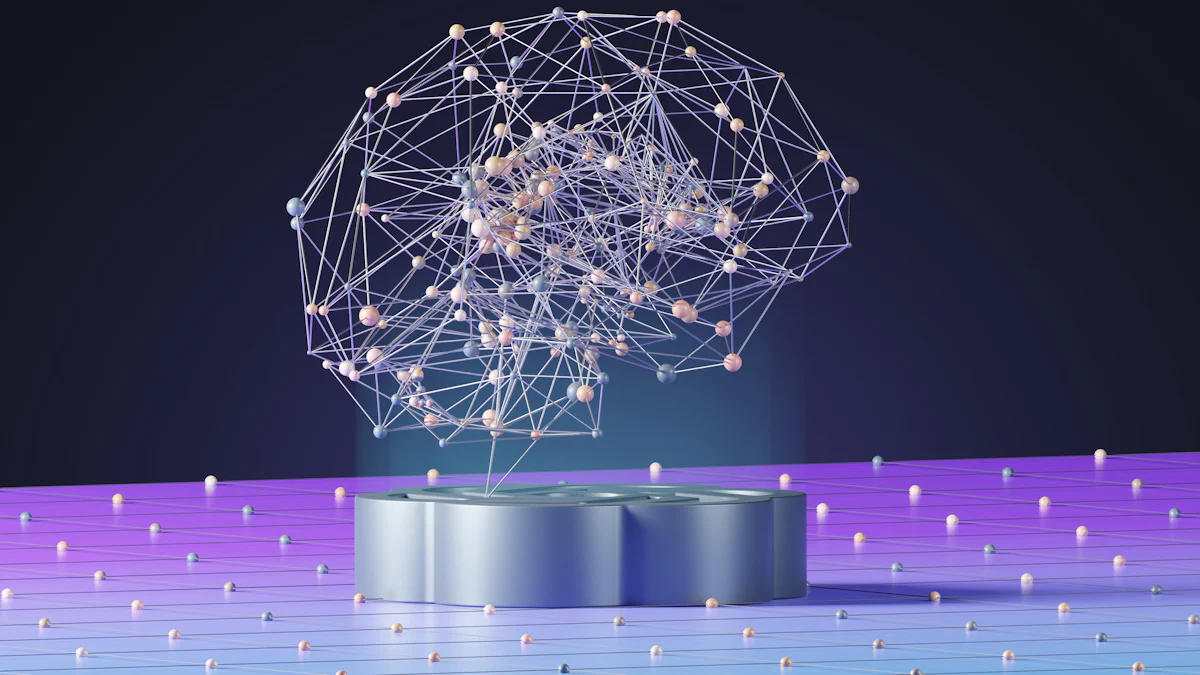
Real-World Use Cases
Image and Text Matching
One of the most compelling applications of OpenAI CLIP is its ability to match images with their corresponding textual descriptions. This capability is particularly useful in scenarios where visual data needs to be categorized or retrieved based on natural language queries. For instance, in digital asset management systems, users can search for specific images using descriptive text, making it easier to locate and organize visual content. This feature is also beneficial in social media platforms, where users can tag and search for images using natural language, enhancing the overall user experience.
Content Moderation
Content moderation is another area where OpenAI CLIP excels. By understanding the semantic relationships between images and text, CLIP can assist in identifying inappropriate or harmful content. For example, it can flag images that contain offensive material based on their textual descriptions, helping platforms maintain a safe and respectful environment. This automated approach to content moderation not only improves efficiency but also reduces the reliance on human moderators, who can then focus on more complex cases.
Visual Search Engines
OpenAI CLIP has revolutionized visual search engines by enabling more intuitive and accurate search capabilities. Traditional image search engines rely heavily on metadata and tags, which can be limiting and often inaccurate. With CLIP, users can perform searches using natural language descriptions, resulting in more relevant and precise search results. This is particularly useful in e-commerce, where customers can find products by describing them in their own words, leading to a more seamless shopping experience.
Industry Impact
Media and Entertainment
In the media and entertainment industry, OpenAI CLIP has opened up new possibilities for content creation and curation. For instance, video streaming services can use CLIP to recommend content based on the visual and textual preferences of users. Additionally, film and television studios can leverage CLIP to organize and retrieve visual assets more efficiently, streamlining the production process. The ability to understand and associate images with natural language descriptions also enhances the capabilities of creative tools, allowing artists to generate and manipulate visual content in innovative ways.
E-commerce
The e-commerce sector stands to benefit significantly from the integration of OpenAI CLIP. By enabling more effective visual search and product recommendations, CLIP enhances the customer shopping experience. Shoppers can describe what they are looking for in natural language, and the system can accurately retrieve matching products. This not only improves customer satisfaction but also increases conversion rates. Furthermore, CLIP can assist in automating product categorization and tagging, reducing the manual effort required to manage large inventories.
Healthcare
In healthcare, OpenAI CLIP can be utilized to improve diagnostic and treatment processes. For example, medical professionals can use CLIP to match medical images, such as X-rays or MRIs, with relevant textual descriptions, aiding in diagnosis. This capability can also be extended to medical research, where researchers can search for specific visual data using descriptive language, accelerating the discovery of new treatments and therapies. The ability to efficiently learn visual concepts from natural language supervision makes CLIP a valuable tool in the medical field.
Advantages and Limitations
Strengths of CLIP
Versatility and Flexibility
One of the most remarkable strengths of OpenAI CLIP is its versatility and flexibility. Unlike traditional vision models that often require extensive labeled datasets and are limited to specific tasks, CLIP can generalize across various domains. This means it can handle a wide range of applications without needing task-specific training. For instance, CLIP can be used for image classification, content moderation, and visual search engines—all with minimal adjustments. Its ability to understand and interpret both images and text makes it a powerful tool for multi-modal machine learning tasks.
Performance and Accuracy
OpenAI CLIP excels in performance and accuracy, particularly in real-world scenarios. Its zero-shot learning capability allows it to classify images into categories it has never seen before, using only natural language descriptions. This is a significant advantage over traditional models, which typically require large amounts of labeled data to achieve similar levels of accuracy. CLIP’s efficient training approach, leveraging a massive dataset of 400 million image-text pairs, ensures high performance while reducing the need for extensive labeled datasets. This makes it quicker and more cost-effective to fine-tune for specific tasks.
Challenges and Limitations
Computational Requirements
Despite its many strengths, OpenAI CLIP does come with some challenges, particularly in terms of computational requirements. Training a model of this scale demands substantial computational resources. The estimated cost for training CLIP on AWS was around 1 million US dollars, highlighting the significant investment needed. Additionally, deploying CLIP for real-time applications can be resource-intensive, requiring powerful hardware to maintain performance. This can be a barrier for smaller organizations or those with limited computational resources.
Ethical Considerations
Another critical aspect to consider is the ethical implications of using OpenAI CLIP. While the model’s ability to understand and generate associations between images and text is impressive, it also raises concerns about potential misuse. For example, CLIP could be used to generate misleading or harmful content, or to invade privacy by identifying individuals in images without their consent. Moreover, the model’s reliance on internet data for training means it can inadvertently learn and propagate biases present in the data. Addressing these ethical considerations is crucial for responsible deployment and use of CLIP in real-world applications.
Future Directions and Research
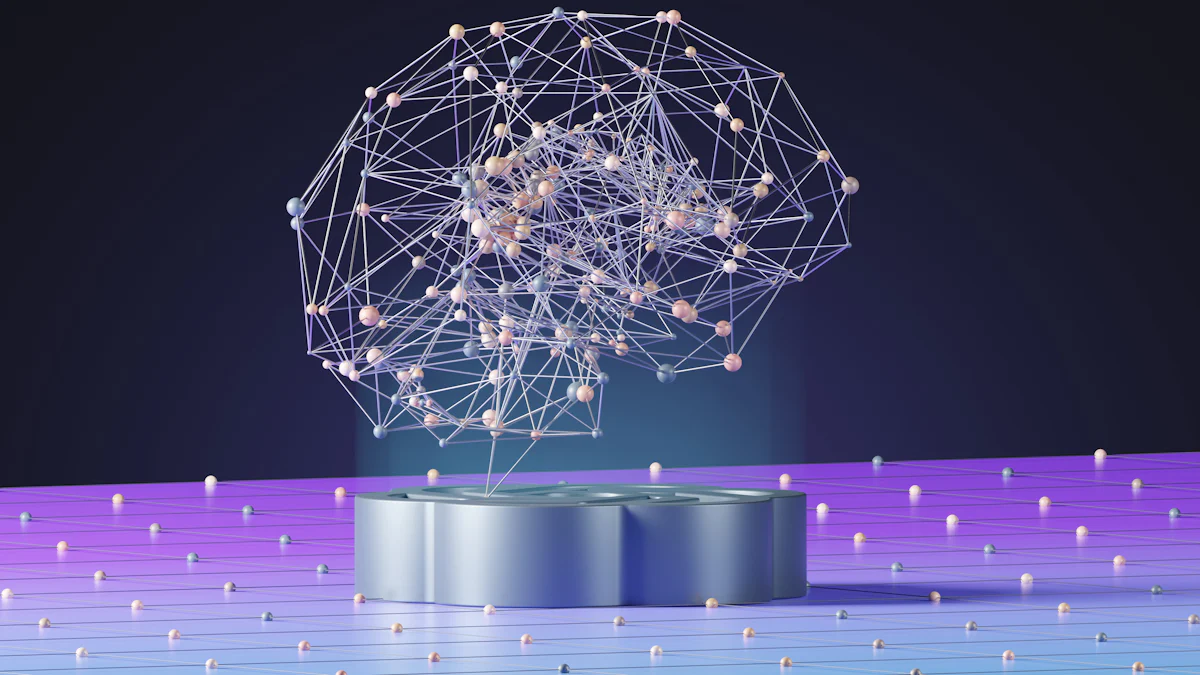
Ongoing Research
Enhancements and Improvements
The continuous evolution of the OpenAI CLIP model is a testament to the relentless pursuit of excellence in AI research. Researchers are actively working on several enhancements to improve the model’s performance and capabilities. One area of focus is refining the contrastive learning techniques to further boost the accuracy and robustness of the embeddings. By experimenting with larger and more diverse datasets, the goal is to minimize biases and enhance the model’s ability to generalize across various domains.
Another significant improvement involves optimizing the computational efficiency of CLIP. Given the substantial resources required for training and deployment, researchers are exploring ways to reduce the model’s footprint without compromising its performance. Techniques such as model pruning, quantization, and distillation are being investigated to create lighter versions of CLIP that can be more easily deployed on resource-constrained devices.
New Applications
The versatility of OpenAI CLIP opens up a plethora of new applications across different industries. For instance, in the field of autonomous vehicles, CLIP can be used to enhance object recognition and scene understanding, enabling safer and more reliable navigation. In the realm of augmented reality (AR) and virtual reality (VR), CLIP’s ability to seamlessly integrate visual and textual data can lead to more immersive and interactive experiences.
Additionally, the healthcare sector stands to benefit from new applications of CLIP. Beyond diagnostics, CLIP can assist in medical education by providing detailed visual explanations of complex medical procedures and conditions. This can be particularly useful for training medical professionals and improving patient understanding of their treatments.
Potential Developments
Integration with Other Technologies
The future of OpenAI CLIP lies in its integration with other cutting-edge technologies. Combining CLIP with natural language processing models like GPT-4 can result in more sophisticated AI systems capable of generating detailed and contextually relevant descriptions of visual content. This integration can enhance applications such as automated content creation, where AI-generated images and text can be used to produce high-quality marketing materials, educational content, and more.
Moreover, integrating CLIP with advanced vector databases like PingCAP’s TiDB can significantly improve the efficiency of data retrieval and analysis. TiDB’s advanced vector database features, such as efficient vector indexing and semantic searches, can complement CLIP’s capabilities, enabling faster and more accurate searches across vast datasets. This synergy can be particularly beneficial in fields like e-commerce, where quick and precise product searches are crucial for enhancing the customer experience.
Expanding Capabilities
As the AI landscape continues to evolve, expanding the capabilities of OpenAI CLIP remains a priority. One potential development is the extension of CLIP’s zero-shot learning capabilities to more fine-grained tasks. While CLIP excels at broad categorization, there is ongoing research to improve its performance on tasks that require detailed differentiation, such as identifying specific species of plants or animals.
Another exciting avenue is the exploration of multi-modal learning, where CLIP can be combined with other sensory data, such as audio or haptic feedback. This can lead to the creation of AI systems that have a more holistic understanding of the world, opening up new possibilities for applications in robotics, human-computer interaction, and beyond.
PingCAP’s TiDB and AI Integration
TiDB’s Role in AI Applications
Advanced Vector Database Features
PingCAP’s TiDB database is a powerful ally in the realm of AI applications. One of its standout features is its advanced vector database capabilities. These features are specifically optimized for AI workloads, enabling efficient handling of high-dimensional data. TiDB supports semantic searches, which allow AI models to retrieve and analyze data based on meaning rather than just keywords. This is particularly useful in applications like image recognition and natural language processing, where understanding context is crucial.
Moreover, TiDB’s horizontal scalability ensures that it can handle large volumes of data without compromising performance. This makes it an ideal choice for AI applications that require processing vast amounts of information in real-time. The ability to scale out by simply adding more nodes allows businesses to grow their AI capabilities without facing bottlenecks.
Efficient Vector Indexing
Efficient vector indexing is another critical feature of TiDB that enhances its utility in AI applications. Vector indexing involves organizing data in a way that allows for rapid retrieval based on similarity. This is essential for tasks like image and text matching, where the goal is to find the most relevant results quickly.
TiDB employs state-of-the-art indexing techniques to ensure that searches are both fast and accurate. For instance, in recommendation systems, TiDB can store and index user interaction data, enabling the AI model to provide personalized recommendations in real-time. This capability was demonstrated by ShareIt, which leveraged TiDB and TiKV to power its AI workflow and recommendation system, resulting in seamless online storage interactions.
Case Studies and Success Stories
Client Testimonials
PingCAP’s commitment to innovation and customer satisfaction is reflected in the success stories of its clients. Companies like CAPCOM, Bolt, and ELESTYLE have praised the flexibility and performance of the TiDB database. These testimonials highlight how TiDB’s advanced features have enabled them to overcome complex data challenges and achieve their business goals.
For example, CAPCOM utilized TiDB to support its gaming infrastructure, ensuring high availability and strong consistency for millions of users worldwide. Bolt, a leading transportation platform, leveraged TiDB to manage its real-time data processing needs, resulting in improved ride-matching algorithms and enhanced user experience. ELESTYLE, an e-commerce platform, benefited from TiDB’s efficient vector indexing, which streamlined product searches and boosted customer satisfaction.
Real-Time Reporting and Analytics
Real-time reporting and analytics are crucial for businesses looking to make data-driven decisions. TiDB excels in this area by providing robust support for Hybrid Transactional and Analytical Processing (HTAP) workloads. This means that businesses can perform real-time analytics on transactional data without the need for separate systems.
One notable case is a financial services company that implemented TiDB to enhance its real-time reporting capabilities. By integrating TiDB with its AI models, the company was able to generate up-to-the-minute insights into market trends and customer behavior. This allowed for more informed decision-making and a competitive edge in the fast-paced financial sector.
In summary, PingCAP’s TiDB database plays a pivotal role in AI applications by offering advanced vector database features and efficient vector indexing. The success stories of clients like CAPCOM, Bolt, and ELESTYLE underscore the transformative impact of TiDB on their operations. Whether it’s powering recommendation systems or enabling real-time analytics, TiDB continues to be a cornerstone of innovation in the AI landscape.
In this guide, we’ve delved into the intricacies of OpenAI’s CLIP model, exploring its groundbreaking contributions to AI technology. CLIP’s ability to bridge the gap between computer vision and natural language processing marks a significant advancement in the field. For those eager to dive deeper, further exploration of OpenAI’s work is highly encouraged. Additionally, PingCAP remains committed to driving innovation in AI and database management, exemplified by the robust capabilities of the TiDB database.
See Also
Enhancing Semantic Capabilities with Azure OpenAI and TiDB Vector Search
Creating RAG with Jina.AI Embeddings API and TiDB Vector Storage
Advancing Your Game: A Developer’s Handbook to AI in Gaming
Constructing a GraphRAG from Wikipedia Page with DSPy, OpenAI, and TiDB Vector Database